Project - 2022
De Bijenkorf web filter redesign
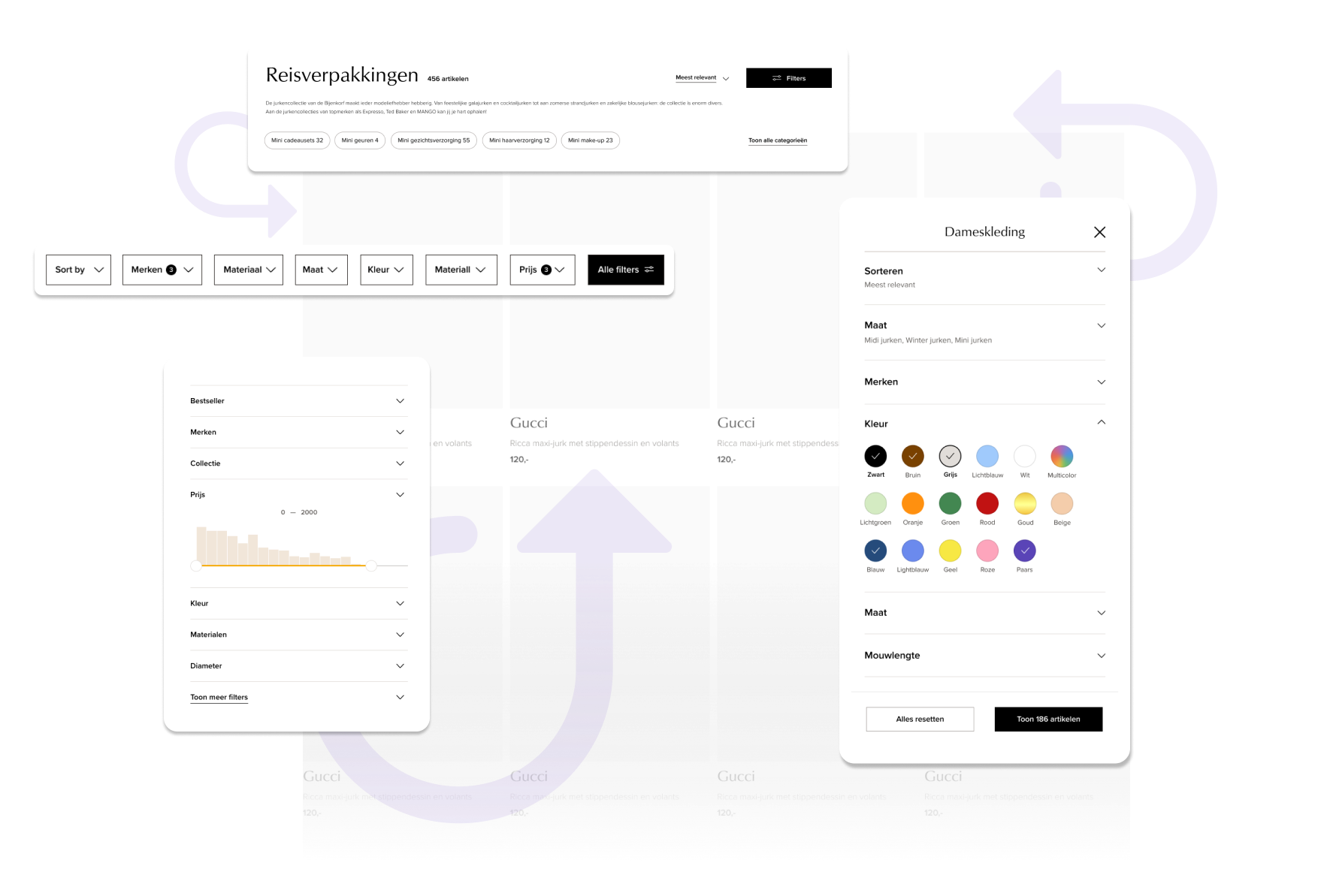
Background
The filtering capability is one of the most critical components for the success of any e-commerce business. At De Bijenkorf we decided to revamp the existing filter, which was deemed outdated and underperforming.
I was tasked with the filter redesign, with the ambitious goal of increasing the conversion rate by 3%.
The filtering capability is one of the most critical components for the success of any e-commerce business. At De Bijenkorf we decided to revamp the existing filter, which was deemed outdated and underperforming.
I was tasked with the filter redesign, with the ambitious goal of increasing the conversion rate by 3%.
The business goal
The user goal
My Role
- Increase the filter conversion rate by 3%.
The user goal
-
Make it easier for users to find the perfect product.
My Role
- User research
- UX design
- UI design
- Data analysis
What did I do?

Identifying Customer Problems
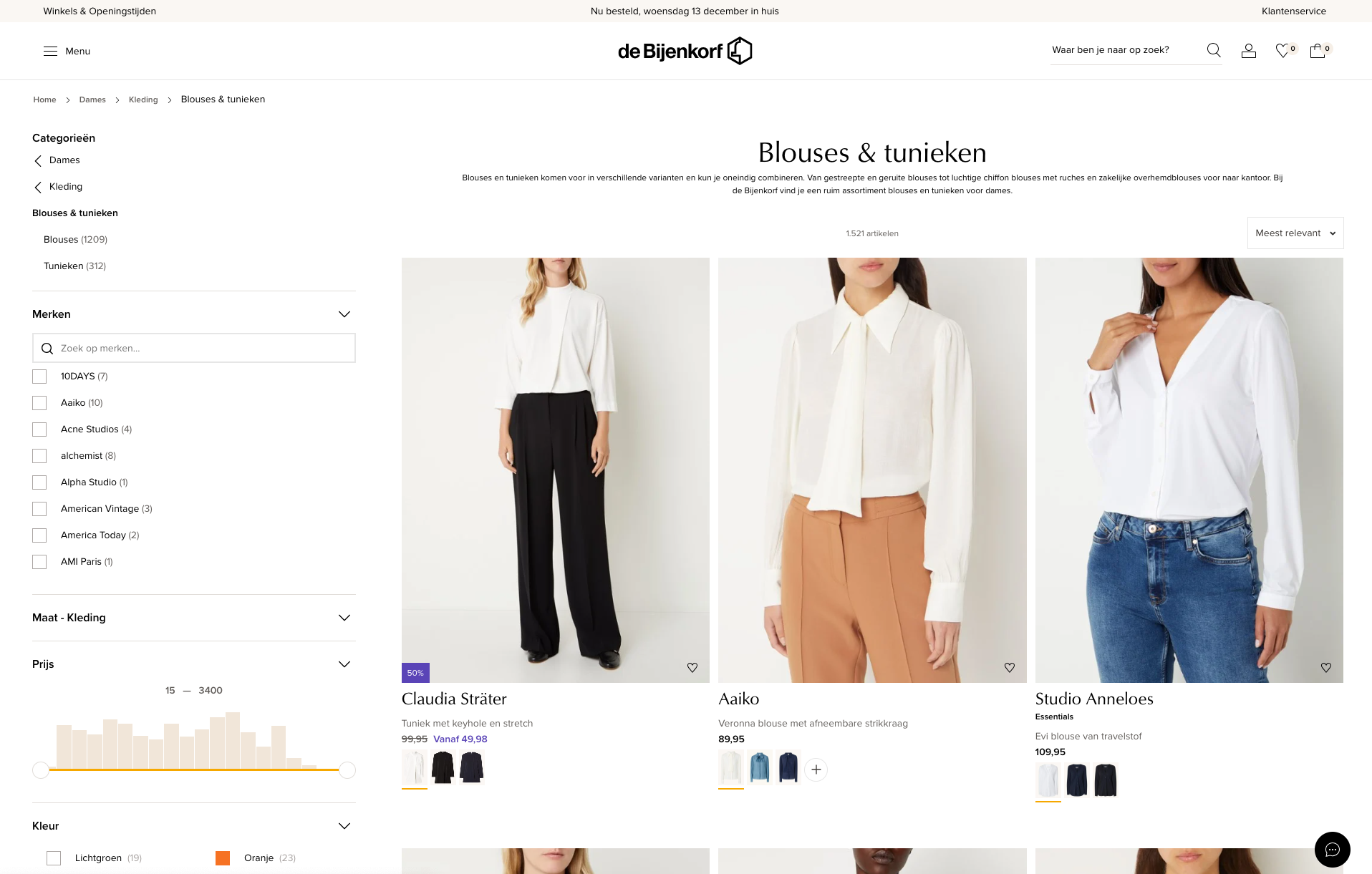
I identified the customer problems based on data, user feedback, Baymard & Nngroup research and our previous research insights:
Main problems:
- Difficulty in locating previously selected filters.
- Long waiting times between selecting different filters.
- The filter results are not personalized
Prioritize Problems
Besides the mainly problems, there are more in current filter. Clearly, not all of these can be addressed at once. Therefore, I categorized these problems into three groups to create a well-defined prioritization plan.

How to measure success?
Before I start to work on the task, I always defined with stakeholders about how we measure success, we decided together to use A/B testing and online survey to measure success from business perspective and user perspective :
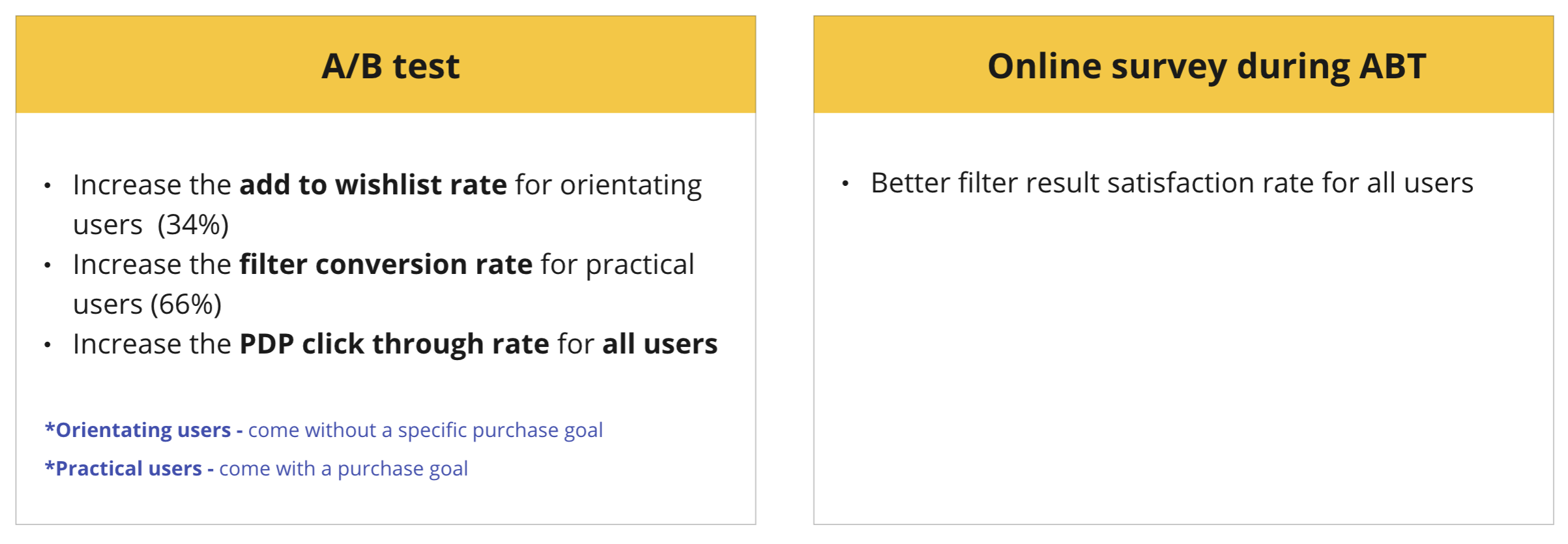
What are the user needs/goals in using filter?
I can imagine that there are different needs based on the different scenarios in using filter, so I conducted a focus group discussion to uncover these questions below:
- What are the scenarios when users use filters?
- What are the User Goals in these scenarios?
- What are the User Needs in each scenario?
This is the summary from the focus group discussion:
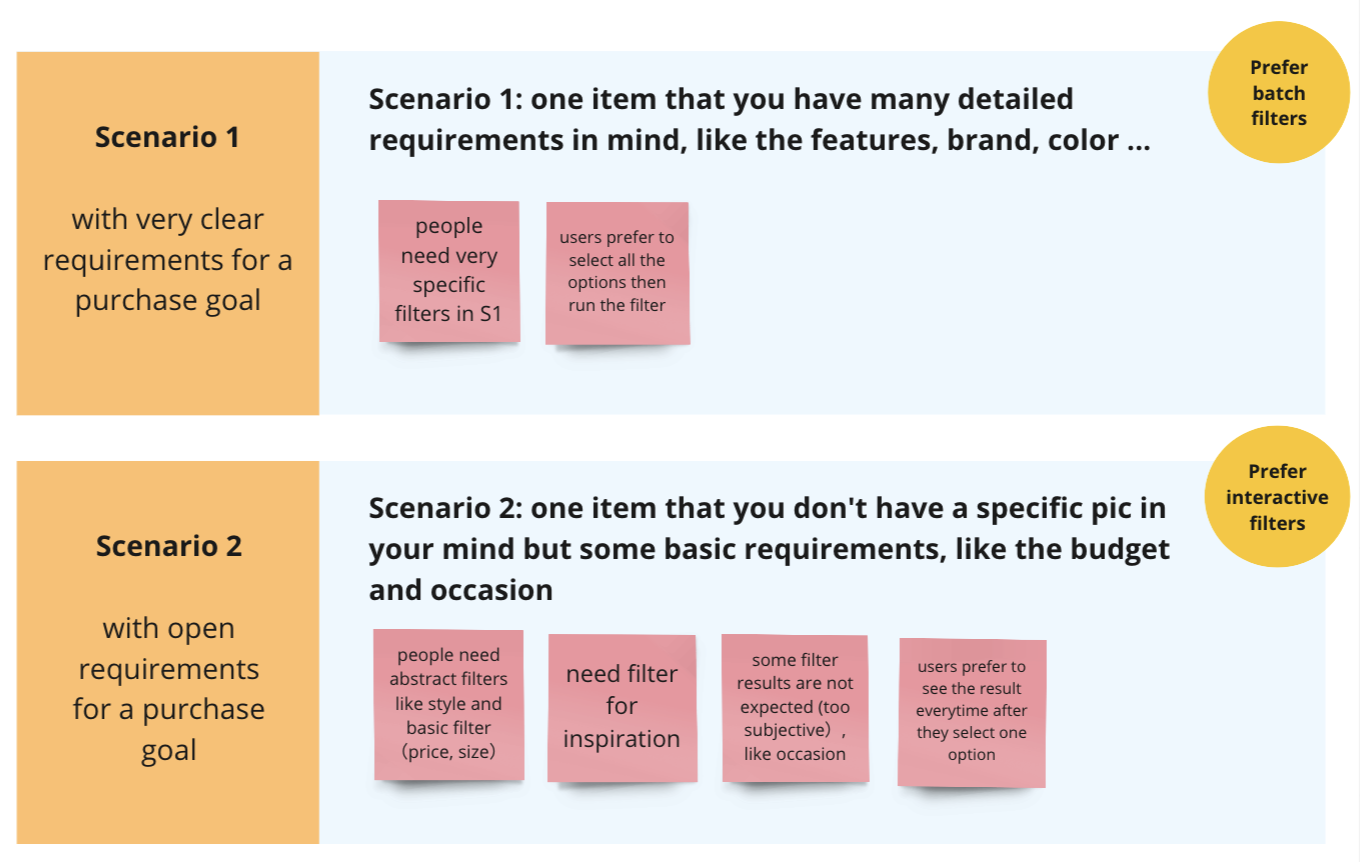
Design Decisions Based on Data
With varying user requirements across different scenarios, the challenge is to determine which requirements to prioritise into the design.
Based on the data, there are 66% users come with a clear purchase goal (scenario 1 & scenario 2) and my focus group discussion and Nielsen Norman group research both indicate their preference for batch filters ( users adjust multiple filters before the results of the compound query are returned ).
Furthermore, the "batch filters" are also used in the current mobile version, from the data that we know it performs better than the interactive filters on desktop ( The results returned after each filter value is specified ). Based on these findings, the decision was made to integrate "batch filters" into the redesign.
Design for Experiment
The Hypothesis:
By updating the filter design, users can find more relevant products, leading to an increase in the conversion rate.
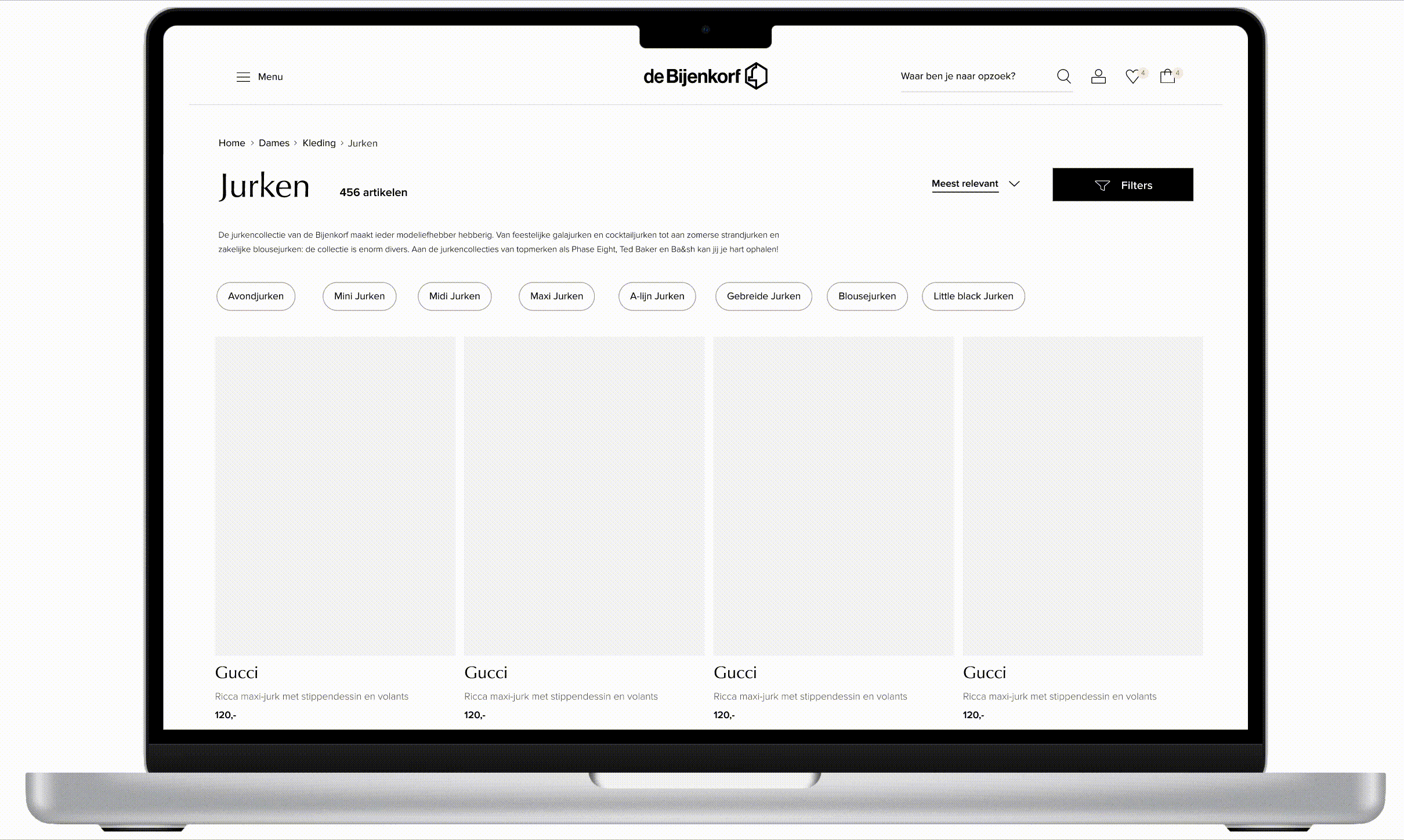
This is a GIF
The A/B testing scope
Test duration
2 weeks
PLP Views
Control: 913515
Variant: 947774
Test duration
2 weeks
PLP Views
Control: 913515
Variant: 947774
The set up
A/B distibution
50/50
Sessions in using filter
Control: 55097
Variant: 35319
A/B distibution
50/50
Sessions in using filter
Control: 55097
Variant: 35319
The result
The finding
What is the reason that the variant group did not perform as well as expected? After I looked into the details, I found that the variant group had lower filter usage but a higher conversion rate in the sessions, so the low filter usage led to a negative impact, and it is probably because of the filter button visibility is low.
![]()
- Click-through rate in the variant group was slightly lower than the control group.
- Conversion rates remained almost the same.
The finding
What is the reason that the variant group did not perform as well as expected? After I looked into the details, I found that the variant group had lower filter usage but a higher conversion rate in the sessions, so the low filter usage led to a negative impact, and it is probably because of the filter button visibility is low.
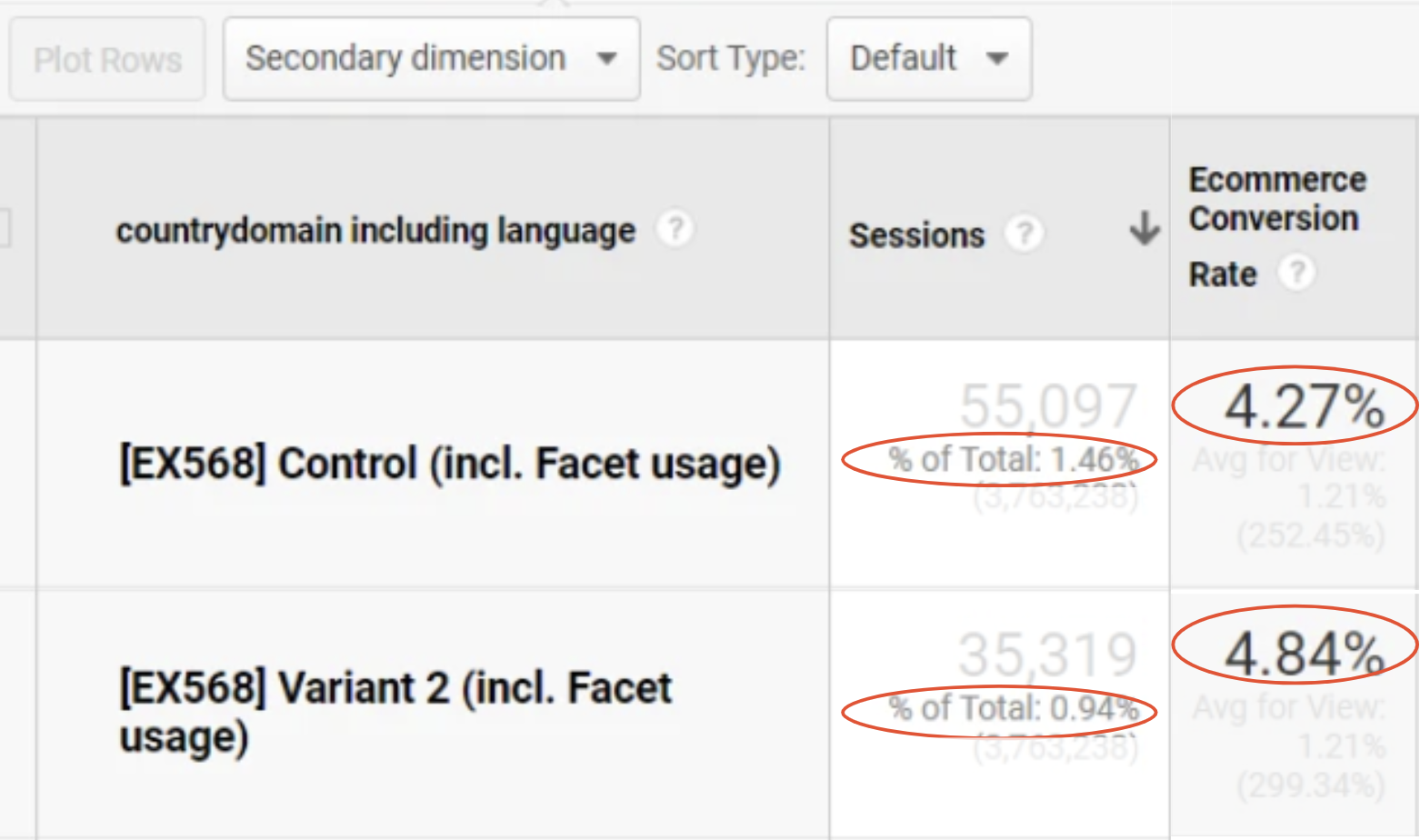
Iteration and Outcome
The visibility of the filter button is low was identified as a potential issue. In the iteration, I integrated both batch and interactive filters, providing users with easy access to the most frequently used filters. But still keep the batch filtering for these users who prefer it.
Iteration
![]()
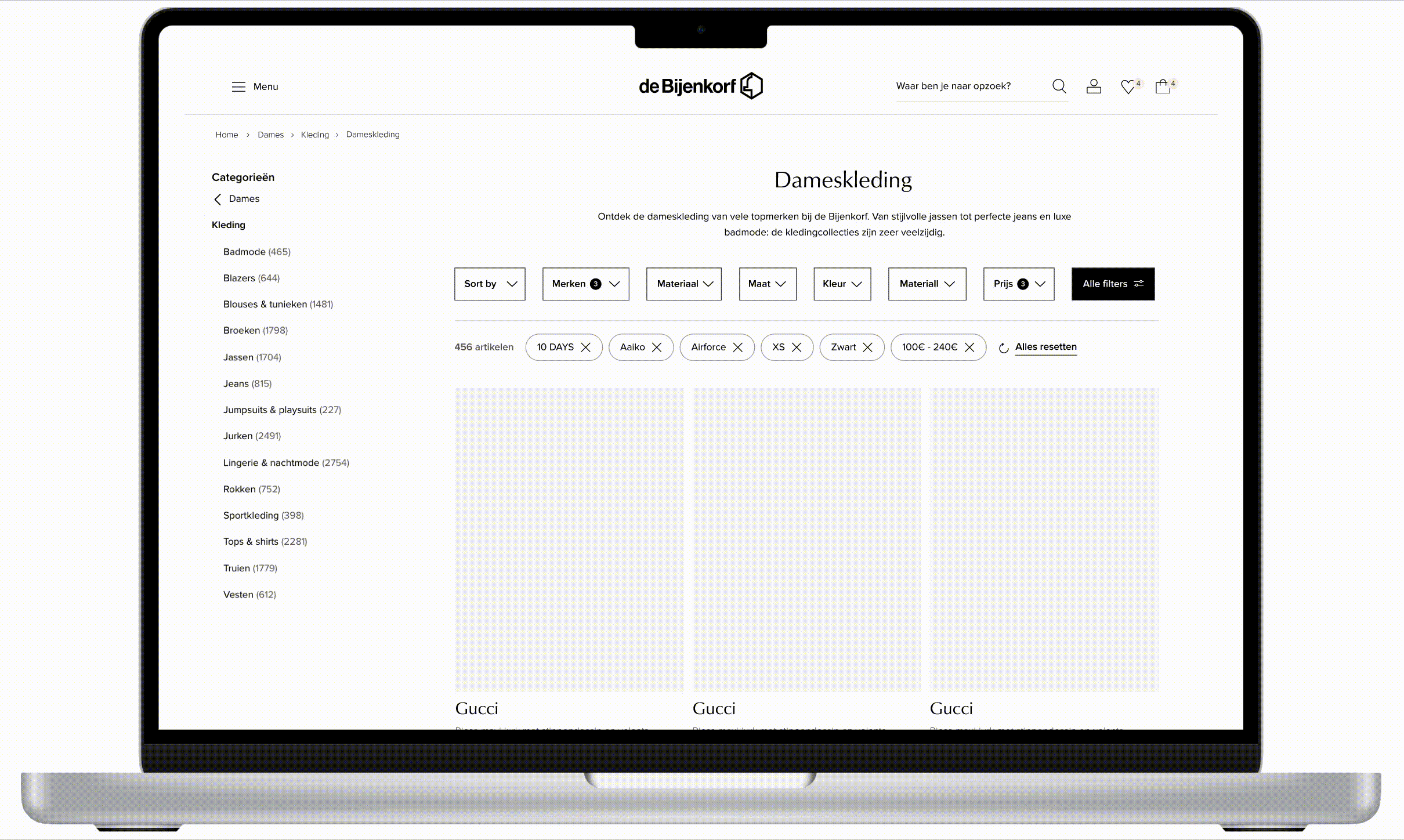
This is a GIF
This filter still under the A/B test now. I will contiue update the following precess.
Learnings
Every experiment serves as an opportunity for learning, and even a failed experiment can be incredibly valuable when we uncover the reasons behind its failure.
I like to view each experiment as a detective story 🕵️ . The primary metrics represent the outcomes, and the meticulous data tracking reveals valuable evidence hided in seemingly unremarkable corners 🔍.
B